Table of Contents
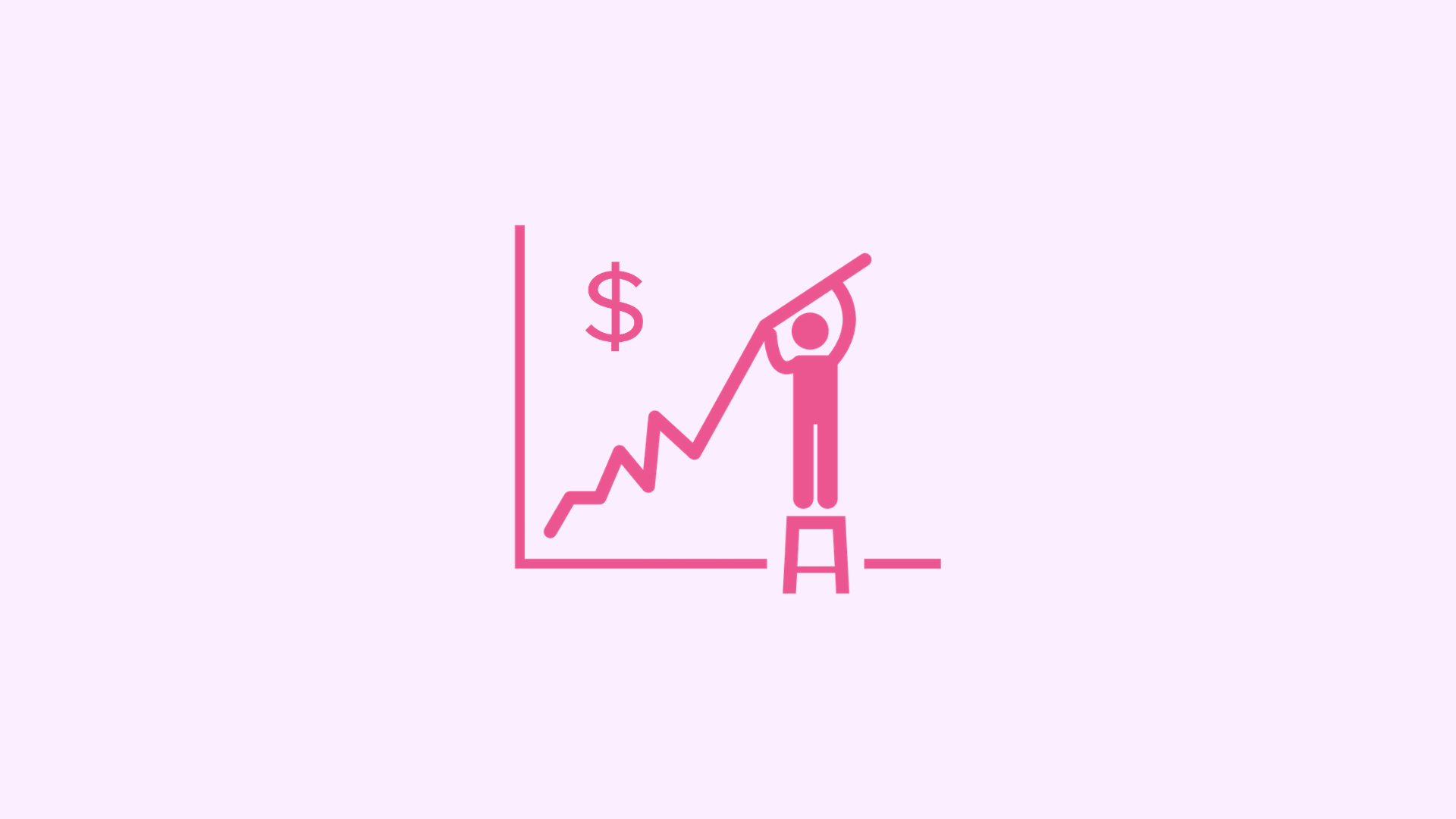
Financial forecasting models are used to predict financial outcomes within a specified area of your business, like recurring revenue or payroll. These models then feed into the overall financial model for your SaaS business.
Adopting this approach provides you with invaluable insights into your subscription-based business, helping you calculate costs, improve budgeting, and allocate resources.
In this article, we’ll have a closer look at five different forecasting methods and present examples of use cases.
Try Baremetrics for free to learn more about how your company could benefit from financial modeling.
What Is Financial Forecasting?
Financial forecasting involves predicting an organization's financial outcomes. Access to a prognosis helps decision-makers create meaningful strategies and make critical decisions about corporate development.
Of course, revenue is one of the most coveted numbers that most businesses want to predict. However, financial forecasting can concern any business area, such as payroll, fixed costs, variable costs, income statements, balance sheets, and capital expenditures.
Many businesses try to create forecasts using Excel but often find that to create thorough financial analysis, financial planning, and long-term budgeting, they need something more advanced.
Read more: How to Build a Financial Model
Here are five financial forecasting models to help you drive business growth:
1. Top-Down Financial Forecasting Models
This model can be handy when you want to evaluate a new opportunity and have no historical data to base your predictions on.
A top-down forecasting model can use the size of a new market as a point of departure and then make a forecast by estimating how much market share your business will be able to grab.
A top-down approach is primarily helpful in the initial phase when you want to evaluate new growth opportunities.
Read more: The Best Financial Modeling Software for SaaS in 2021
2. Bottom-Up Financial Forecasting Models
If you have access to historical sales data or financial statements, it makes sense to approach forecasting from the bottom up. Then, you can use your existing sales numbers and cash flow statements as input for calculating future scenarios.
This method will usually be more accurate and more detailed since you are working with actual numbers, so you reduce the assumptions.
Interested in how your business can benefit from a modern tool for financial modeling? Try Baremetrics for free!
3. Delphi Forecasting Models
The Delphi method is a model where you get your forecast from a group of experts, leveraging a facilitator and continuously collaboratively iterating on hypothesis and analysis to reach a consensus opinion.
A series of questionnaires form the basis of this process, where every questionnaire builds on the previous iteration. This is an efficient way to make sure the entire group gets access to all information.
4. Correlation-Based Forecasting Models
Another way to look at financial forecasting is to identify correlating variables and track how they follow each other. This is a widespread financial forecasting model.
This way of predicting financial outcomes can help decision-makers understand make forecasts based on the relationships between prices and costs, supply and demand, and other factors that affect each other.
5. Statistical Forecasting Models
Statistical models (also called quantitative forecasting models) create relationships between the findings of other disciplines. This approach often uses Gaussian distribution analysis to fit financial inputs and attempts into a classic standard distribution curve.
This can help you figure out how your operation compares to similar businesses, and you can use this method for benchmarking., growth rate, profitability, and decision-making.
Read more: The New Era of SaaS Forecasting
Power Laws in Financial Forecasting
Power laws represent a complex and challenging analytic model that is sometimes used in financial forecasting models. They are mathematical functions describing proportional movements between assets.
Power laws are prevalent in the stock market and corporate finance, where they are popular because they can quickly highlight and break down specific momentum trends.
The knowledge derived from using the Power laws approach can be an excellent guide for resource allocation, capital purchases, marketing, and other types of similar internal investments.
Interested in learning more about how you can grow your SaaS company’s revenue?
Read our article: How To Improve Revenue Growth
How Baremetrics Can Help!
Financial forecasting models attempt to predict a business's financial future and estimate its potential. Unlike working with a financial analyst, the results are never 100% accurate.
However, financial forecasts are essential in budgeting and growth planning and when making financial decisions.
Baremetrics optimizes forecasting with a broad range of real-time metrics for churn, MRR, cost of acquisition, business valuation, and other key performance indicators that pertain to financial performance.
The result? SaaS and subscription-based companies like yours make more efficient business decisions and create profitable growth strategies. Investing in this forecasting software is a great way to maximize resource allocations' impact on your company’s bottom line.
Building a comprehensive, growth-focused financial model takes some work and effort. But it’s an investment that is worthwhile since the benefits of reliable financial data predictions are critical to growing your business.
Try Baremetrics for free to learn more about how your company could benefit from solid financial modeling.
FAQs
-
How does the Baremetrics financial forecasting application help in making accurate predictions?
Baremetrics' financial forecasting application helps make accurate predictions through features such as data integration, historical data analysis, scenario modeling, intelligent algorithms, and real-time updates. It provides clear visualizations of forecasted outcomes. For more information visit: https://baremetrics.com/features/forecasting
-
How can financial forecasting software benefit my business?
Financial forecasting software provides several benefits for businesses, including fine-tuning business plans, generating budget templates, tracking historical budgets, comparing projections with actual figures, consolidating budgets across departments, planning for different scenarios, and monitoring budgeting process performance.
Learn about financial forecasting software for SaaS businesses.
-
How can financial forecasting tool aid in budget planning and decision-making processes?
A financial forecasting tool aids budget planning and decision-making by providing accurate projections, enabling scenario analysis, facilitating resource allocation, monitoring performance, supporting collaboration, and assisting in long-term planning. -
What are the key features to look for when selecting financial forecasting software?
When selecting financial forecasting software, key features to look for include:
- Accurate financial projections
- Flexible scenario modeling
- Integration with financial data sources
- Historical data analysis
- Collaborative forecasting
- Real-time tracking and reporting
- Integration with budgeting and planning
- Scalability and customization.
Considering these key features, you can select financial forecasting software that meets your business requirements and empowers you to make informed financial decisions. -
Can you recommend any software for financial forecasting that integrates with accounting systems?
Baremetrics is an essential tool for SaaS financial forecasting software. Baremetrics offers seamless integration with QuickBooks Online and Xero, allowing for the synchronization of financial data between the software and these accounting platforms. This integration ensures accurate and up-to-date financial forecasting and analysis.